Turning cities into data
Lidar, mapping informal communities, and the possibilities of structured data
Informal settlements, or favelas, are common in Brazilian cities. These communities, home to millions, are completely disconnected from basic infrastructure. They were never given sewage systems. Nor were they given access to power grids. And what infrastructure residents do have, they typically had to build themselves.
There are now efforts to reintegrate these places with the cities that surround them, but planners have no idea how they're laid out. Authorities never provided things like roads, there's a lack of topographical data, and the lack of information makes it difficult to bring in the proper infrastructure now.
Enter the Favelas 4D project. Researchers are setting out to solve this problem using Lidar to scan unmapped areas and create high-fidelity 3D maps.
Building cityscapes with Lidar
Lidar works by using a device to pulse light into the surrounding environment. The light reflects off nearby objects, returning back to sensors on the device. These sensors then calculate the time it takes for each pulse to make the round trip. The longer the travel time, the farther away the object is. The resulting data is a series of points with x, y, and z coordinates (called a point cloud) relative to the Lidar device.
Afterwards, there’s an additional processing step to generate something a human would recognize as a cityscape. For this part, the Favelas 4D team used Random Sample Consensus to transform discrete points into planes (i.e. facades and streets). They then used a nearest-neighbor approach to group planes into street scenes.
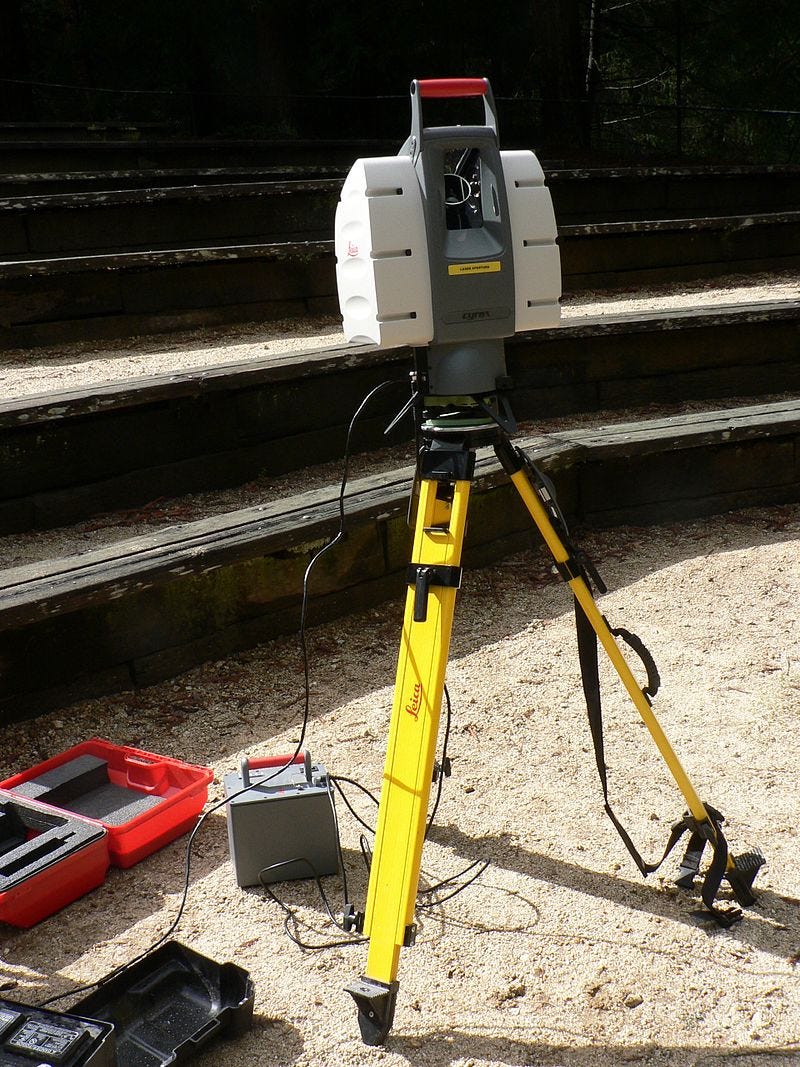
If that’s all a bit confusing, think of this as sonar for a submarine or echolocation for a bat (but with light).
Lidar in our pockets
One of the benefits of the Favelas 4D approach is that it’s going to get easier to do. While the researchers used purpose-made equipment, Lidar equipped mobile devices would serve as well.
iPhone 12s and above (in Pro or Max) all come Lidar-equipped. This is partially to improve photo quality, but there's also a growing number of Lidar-enabled apps. Augmented reality experiences for interior design and renovation are increasingly common. Moreover, Apple is supporting developers’ work with Lidar data via it’s Room Plan API. Outside the home, iPhone-equipped Lidar performs well compared to specialized equipment for surveying.
A killer hardware feature
Lidar will become standard on mobile. It's also likely to be common on whatever other forms consumer hardware takes in the future. That might include wearables. More likely, though, are Lidar-equipped personal drones that follow their owners around like Navi from Ocarina of Time.
As Lidar becomes ubiquitous, point cloud data will become more common and easier to produce. That could result in new standards for architectural and planning documentation. Imagine city records with detail down to which trees got blown over in the last big storm. More exciting though, are the other technologies we can apply on top of structured data.
On the consumer side, firms are already working on interior design where the input is a scan of a room and a prompt like “mid-century modern”. But there’s no reason we can’t apply similar techniques to larger problems in urban planning.
A large language model, provided with all the relevant context, could optimize transit routes or placement of shelter beds. And this isn’t necessarily a story of machines doing what people can’t, but perhaps doing work in minutes that takes days or weeks to slog through today. Even that could be revolutionary on it’s own.
The Favelas 4D Project is trying to remove a technical barrier to addressing a major policy issue. It’s important work that will hopefully bear fruit for folks in Brazil and the over one billion people in similar conditions worldwide. Technologically, though, there's another story. Ubiquitous Lidar will enable us to turn the physical world into structured data. That data in hand, myriad different possibilities will open up as the cutting edge of machine learning continues marching forward.